Train a model to detect MuSHR cars.
Intermediate Tutorial | Expected duration is 60 minutes
By: Tudor Fanaru & Sean Chen
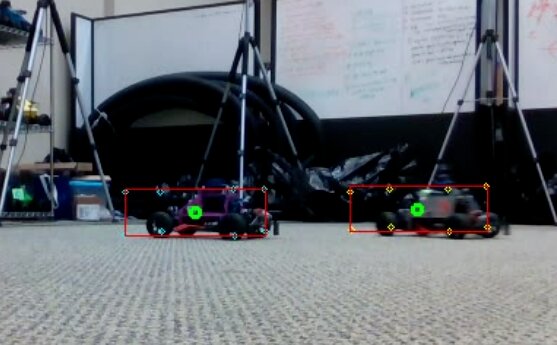
Introduction
Goal
In a multi-agent system, car detection is one of the fundamental processes that must take place in order for cars to make decisions from their oberservations. This applies to collision avoidance & navigation, as well as any other form of cooperative task. This tutorial will provide instructions for producing bounding prisms to label cars based on mocap car pose data, and training a model from your newly labeled data.
Requirements
- Understanding of Linear Algebra
- Complete the quickstart tutorial
- Complete the Intro to ROS tutorial
- Recommended Readings:
Mathematical Explanations
It is important to remember that the sensor measurements from the cars and mocap all have their own frame of reference. In order to combine these into a bounding box within a single car’s camera frame, we need to be able to switch between frames of reference in a computationally effective way. This is done through the use of transforms. There are 4 important frames of reference: the world (where the mocap marker is tracked), the base_link (root of a car’s tf tree), the camera on the car, and the centroid of a car (used to generate a bounding prism). Being able to move between these frames of reference is necessary to derive the true position of a car on a camera frame, and create a corresponding label.
In this example, car 1 is being viewed by car 2’s camera. Let x_T_y mean the transform of x w.r.t. y:
- marker1_T_cam2 = base2_T_cam2 * marker2_T_base2 * world_T_marker2 * marker1_T_world
- center1_T_cam2 = marker1_T_cam2 * base1_T_marker1 * center1_T_base1
marker1_T_world and marker2_T_world are given by the mocap data world_T_marker2 is the inverse of marker2_T_world base_T_cam, marker_T_base, center_T_base are all constants depending on how you set up your system
Bag Creation:
Whether you create your own dataset using your own bag(s), or you use ours, these are the key ROS topics for each car:
- d435 color camera: /carXX/camera/color/image_throttled
- mocap pose: /vrpn_client_node/carXX/pose
- camera parameters: /carXX/camera/color/camera_info
You can download our bag to see how we gathered data, or to follow along the rest of the tutorial with.
Creating Your Dataset:
Now that you have a bag, you will use gen_darknet_label.py to create your labels & dataset in the darknet format. The implementation of YOLOv5 we are using requires the dataset to be in darknet format:
- bounding boxes are represented by (x_center, y_center, w, h)
- each value is in terms of the dimension of the image between 0 and 1
Create a dataset directory, and create two subdirectories: images & labels. Run the gen_darknet_label.py script to populate the directories with images and labels. When finished, your dataset directory should look like this:
dataset/
images/
img_000000.jpg
img_000001.jpg
...
labels/
img_000000.txt
img_000001.jpg
...
You can download our dataset to jump right into the machine-learning aspect of this tutorial.
Labeling Implementation
The entire implementation code can be found at the car pose detection repo.
After importing all the necessary modules and defining constants, the first thing the script will do is process the entire bag looking for the topics listed above. You will want:
- The pose of each car
- The camera frames and camera info from one car
|
|
Next, it will step over each video frame. Since the motion capture data is published at a much higher rate, we must find the mocap data to each video frame. Each message of a rostopic has a timestamp, so we pick the mocap message with the closest timestamp to the video frame’s timestamp.
|
|
Next, we create and use transforms to identify where the other cars’ corners are on the frame. We load in the frame as an OpenCV image. Should you need to debug, you can draw the points of interest on the frame.
|
|
Once we’ve found where each bounding box is, we have to decide whether or not to include it in the dataset. First we reject all labels of cars behind the camera. We also wish to throw out a label when one car is occluding another - we only want the front car to be labeled in this case. Finally we save the image and its label in their corresponding locations.
|
|
Setting Up YOLOv5
First clone the YOLOv5 repo: - https://github.com/ultralytics/yolov5
Now install all the requirements from requirements.txt Now create your train/valid/test files. Each file will contain the location of the images you will use for training (the labels will be automatically grabbed since the directory structure is known ahead of time). We split our dataset in a 70/20/10 ratio for train/valid/test, respectively.
Here are the first 3 lines of our train.txt:
- /mnt/hard_data/carpose/dataset/images/car37_longtrim_000000.jpg
- /mnt/hard_data/carpose/dataset/images/car37_longtrim_000001.jpg
- /mnt/hard_data/carpose/dataset/images/car37_longtrim_000002.jpg
Next create carpose.yaml, which will describe the dataset. Fill in the paths to your train/valid/test files. We only have 1 class: Car.
Here is our carpose.yaml file:
# train and val datasets (image directory or *.txt file with image paths)
train: /home/ugrads/WRK/carpose/src/yolov5/data/train.txt
val: /home/ugrads/WRK/carpose/src/yolov5/data/valid.txt
test: /home/ugrads/WRK/carpose/src/yolov5/data/test.txt
# number of classes in your dataset
nc: 1
# class names
names: ['Car']
This file should be in yolov5/data.
Training Your Model
Now we can begin training our model! YOLOv5 has four different model sizes: S, M, L, and XL. We used the smallest: yolov5s.yaml. The parameters we used were: python train.py –data ./data/carpose.yaml –cfg ./models/yolov5s.yaml –logdir /mnt/hard_data/Checkpoints/carpose/runs/ –workers 0 –img-size 640 –single-cls –device 1 –batch 16 –epochs 10 –weights ‘’
Change the logdir to wherever you want the output to be, this will include model checkpoints, plots, and examples from each epoch. The –workers parameter only worked when set to 0 for us. The –device parameter selects which cuda device to use. You can increase/decrease the batch size based on your GPU, and change the training length (epochs). You can also use previously stored weights by filling in the –weights parameter.
Alternatively, you can download our trained model.